Training Robots in the AI-Powered Industrial Metaverse
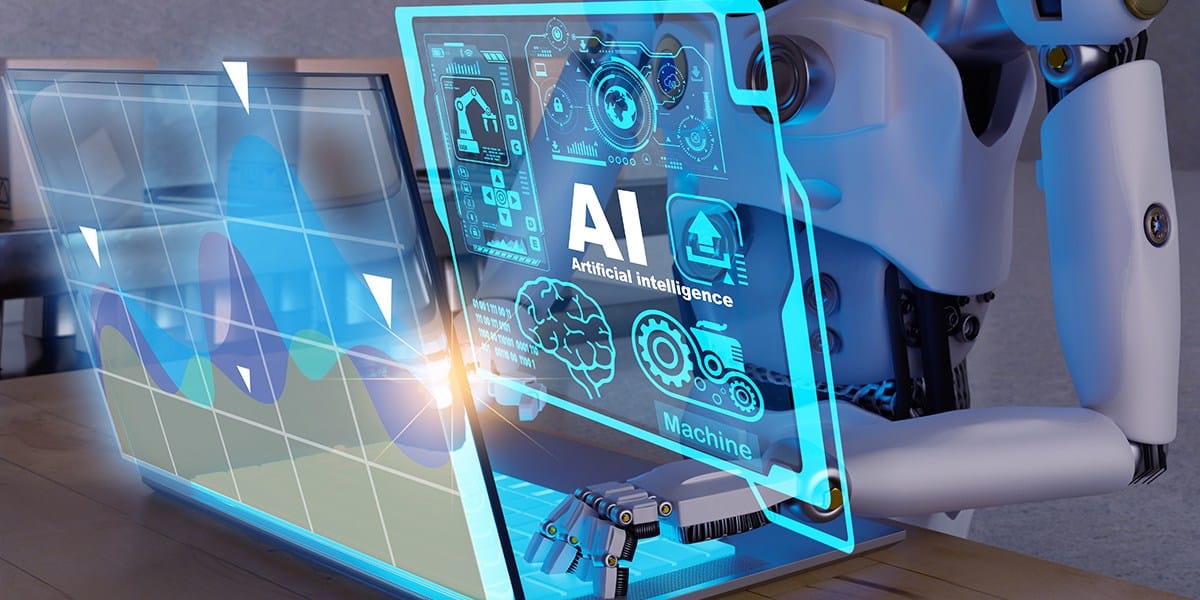
Training for industrial robots has evolved from rigid, traditional methods to embracing immersive environments within the industrial metaverse. These virtual realms leverage simulation, digital twins, and AI to meticulously replicate real-world conditions. The metamorphosis essentially turns industrial metaverse into "virtual classrooms," offering boundless training grounds resembling actual factories and production lines where robots can practice tasks and enhance problem-solving abilities.
This innovative practice, known as "simulation to reality" (Sim2Real), melds virtual training with tangible applications, expediting the learning process remarkably. Tasks that once mandated several days or weeks of manual programming can now be executed within mere hours in these virtual spaces.
Despite being in its nascent phase, the industrial metaverse demonstrates significant potential to revolutionize robot training, bringing an unprecedented level of flexibility.
Franco Filippi, chairman and CEO of the Italian automation provider EPF, remarked on this transformation: "We changed our development strategy from designing entire solutions from scratch to developing modular, flexible components that could be combined to create complete solutions." This shift allows for greater adaptability across different sectors.
Learning By Doing
AI models enhance their effectiveness when exposed to extensive datasets, necessitating hours of robotic operations and human supervision to perfect even a single task. Basic instructions, such as "grab a bottle," can produce diverse results based on variables like the bottle’s shape and environment, causing training to become monotonous and inefficient.
Key to advancing robotics is developing AI models that generalize and succeed in varying environments. Researchers have introduced robot utility models boasting a 90% success rate in executing basic tasks across diverse settings without additional training. These models utilize large language models and computer vision to offer continuous task completion feedback, speeding up learning and eliminating repetitive cycles.
Robotics companies now deploy advanced perception systems that train and generalize capabilities across various domains. EPF, collaborating with Siemens, integrated visual AI and object recognition into its robotics solutions, enabling adaptation to diverse product geometries and environmental conditions sans mechanical adjustments.
Learning By Imagining
AI's progress in robotics faces challenges due to the scarcity of training data. Nonetheless, innovations leveraging digital twins and synthetic data have notably reduced the reliance on expensive traditional methods.