GSK's Advanced Strategies to Tackle Hallucinations in AI-Powered Drug Development
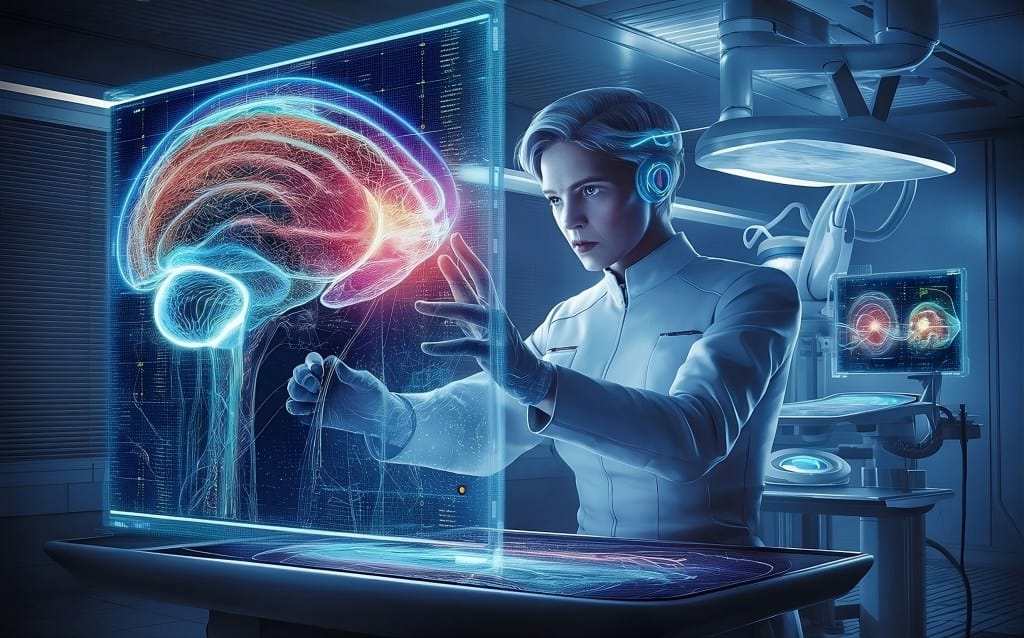
Generative AI is rapidly becoming an essential tool across various sectors, including healthcare. However, with the adoption of this technology, companies like GSK are confronting substantial challenges, primarily related to the reliability of AI outputs. A major concern is "hallucinations," where AI models produce incorrect or misleading information, posing serious risks in fields like drug discovery and healthcare due to the high stakes involved.
The Hallucination Problem in Generative Health Care
In the realm of healthcare, precision and dependability are critical as errors can have profound consequences. This necessitates the need for enhanced measures against hallucinations in large language models (LLMs). GSK, applying generative AI to tasks such as scientific literature review and drug discovery, is actively seeking to minimize these issues.
"These techniques help ensure that agents are 'robust and reliable', enabling scientists to generate actionable insights more quickly," stated Kim Branson, SvP of AI and machine learning at GSK.
Leveraging Test-Time Compute Scaling
Test-time compute scaling enables AI systems to engage more computational resources during the inference phase, facilitating complex operations that reduce hallucinations. This strategy has been transformative for GSK's AI initiatives.
"We’re all about increasing the iteration cycles at GSK — how we think faster," Branson emphasized, highlighting the importance of strategies like self-reflection and ensemble modeling.
According to Branson, the industry is experiencing a "war" over computational efficiency, with organizations competing to lower costs and enhance processes, fueling the effective deployment of AI models.
Strategies for Reducing Hallucinations
GSK employs strategies that necessitate additional computational resources to ensure reliability. These include comprehensive processing steps to verify each output's accuracy and consistency for clinical settings.
Self-Reflection and Iterative Output Review
Self-reflection involves AI models critiquing their responses to enhance quality. In GSK's case, this involves LLMs re-evaluating outputs to identify flaws, thereby improving the insights provided.
"If you can only afford to do one thing, do that," Branson advised, stressing the value of refining AI logic prior to delivering results.
Multi-Model Sampling
This strategy involves deploying multiple LLMs or different configurations of a single model to cross-verify results. It enables more reliable conclusions, despite requiring more computational resources.
"You can get that effect of having different orthogonal ways to come to the same conclusion," explained Branson, illustrating the approach's effectiveness in high-stakes scenarios.
The Inference Wars
The infrastructure capable of handling increased computational loads is pivotal for GSK’s strategies. Companies like Cerebras are advancing technologies that enable efficient inferencing, critical in deploying generative models in healthcare.
"You’re seeing the results of these innovations directly impacting how we can deploy generative models effectively in healthcare," Branson noted.
Challenges Remain
Despite these advancements, scaling compute resources introduces challenges such as longer inference times and increased costs. Nonetheless, GSK deems these as necessary trade-offs for enhancing reliability and functionality.
"As we enable more tools in the agent ecosystem, the system becomes more useful for people," Branson commented, showing the balance between performance and cost.
What’s Next?
GSK is committed to refining its AI solutions, with test-time scaling as a priority. Their roadmap offers insights into integrating accuracy, efficiency, and scalability, addressing current challenges while paving the way for advancements in drug discovery and patient care.